Introduction
Intramuscular fat, also called marbling, in Korean cattle is an important trait that influences the beef quality grading system. Fat accumulation has been shown to be associated with the levels of genes, proteins, and metabolites (Picard et al., 2012; Picard et al., 2015; Segers et al., 2017). In particular, differences in meat quality may be related to changes in muscle metabolism.
Metabolomics is used to detect and quantify metabolites in biological samples, such as fluids, tissues, and cells (Dettmer et al., 2007). Metabolic profiles can be evaluated as output results for biological systems and used to identify potential indicators (Kosmides et al., 2013). Numerous studies have used metabolomics to screen for biomarker (Carrillo et al., 2016; Kennedy et al., 2017; Meale et al., 2017; Williams et al., 2015; Zang et al., 2014). This method has also been used to identify taste compounds in beef meat and to exploration unique biochemical molecules (Carrillo et al., 2016; Yang et al., 2018). Metabolomics has been used alone or in combination with multiplatform methods to elucidate the complex interplay of molecular systems (Tian et al., 2016; Yang et al., 2016). Many metabolomics techniques have been developed, including nuclear magnetic resonance (NMR) spectroscopy, gas chromatography-mass spectrometry (GC-MS), and liquid chromatography-mass spectrometry (LC-MS). These methods can be very useful for rapid analysis of many samples and provide highly sensitive results based on multivariate analysis, pathway analysis, and correlations in food metabolomics (Bartel et al., 2013; Ma et al., 2016; Wei et al., 2018).
Beef taste and palatability are important factors for meat scientists and consumers. Numerous researchers have developed appropriate mechanical procedures for measuring beef taste (Gómez et al., 2014; Jeremiah et al., 2000; Silva et al., 2017). Sweetness, sourness, saltiness, bitterness, and umami tastes are associated with meat chemicals and metabolites, resulting in influence the overall acceptability (Sugimoto et al., 2017; Zou et al., 2018). Therefore, metabolites affecting beef quality attributes provide our understanding of taste mechanisms in beef.
Thus, the objective of this study was to investigate the meat metabolite profiles related to differences in beef quality attributes using NMR spectroscopy.
Materials and Methods
The experimental procedures were approved by the Institutional Animal Use and Care Committee of the National Institute of Animal Science (NIAS) in Korea. Twenty-one beef samples (from cattle approximately 28 months of age) were collected from steer in the NIAS livestock butchery at post-mortem day 1 and then partial stored −80°C such as NMR. Ribeye (longissimus thoracis) samples were taken from the dorsal area of the 13th rib. After slaughter and chilling at 2°C for 1 day, the extent of marbling was determined on the left side of the carcass from the first lumbar vertebra to the last rib using the beef marbling standard (BMS) score according to the Korean Institute for Animal Products Quality Evaluation (KAPE). The carcasses were graded as having lower marbling scores (MSs; 2–5 on the scale of 1–9) with low fat contents (FCs, 13.6± 1.14%) or higher MSs (6–9) with high FCs (18.97±1.45%). Two available beef groups (high-marbled versus low-marbled meat) were chosen based on MSs. Meat samples were frozen using liquid nitrogen and pulverised for metabolomics analysis.
Meat samples (25 mg) were used for 1H-NMR metabolic profiling. Briefly, samples were transferred to 4-mm NMR nanotubes with 25 μL deuterium oxide containing 2 mM 3-(trimethylsilyl) propionic-2,2,3,3-d4 acid sodium salt (TSP-d4; Sigma Aldrich, St. Louis, MO, USA) as an internal standard. The NMR spectra for meat samples were acquired by a 600 MHz Agilent NMR spectrometer (Agilent Technologies, Palo Alto, CA, USA) with a 4-mm gHX NanoProbe for high-resolution magic angle spinning at Pusan National University in Korea. Data were collected at a spinning rate of 2,000 Hz. A Carr-Purcell-Meiboom-Gill pulse sequence was used to reduce the background signals of water and macromolecules in the tissues. The 1H-NMR spectra were measured using 13 μs of a 90° pulse, 0.065 s of bigtau, 2 s of relaxation delay, 1.704 s of acquisition time, and 10 min 20 s of total acquisition time. The TSP-d4 peak at 0.0 ppm was used for reference to calibrate the chemical shifts. Assignment of spectra and quantification of metabolites were accomplished by Chenomx NMR suite 7.1 software (Chenomx, Edmonton, AB, Canada).
The sensory evaluation with minor modifications was conducted in Animal Product Utilization Division of NIAS using the method as described (Cho et al., 2016). The procedure was approved by the Institutional Review Board (IRB) of NIAS (No.11-1390744-000007-01). Panel testing of meats was performed by seven trained researchers (Korean, IRBNIAS). Meat samples were prepared by cutting parallel to the muscle fibre orientation (20×40×10 mm) and scored for color, flavour, juiciness, and tenderness. The sensory tests were graded on numerical scale ranging from 1 (e.g., not beefy, very tough, and very dry) to 7 (e.g., very beefy, very tender, and very juicy).
For the taste analysis of meat samples, an electronic tongue (Astree, Alpha MOS, Toulouse, France) was used with an automatic sample analyser. Taste sensor module was composed of seven sensors (Sensor array #5; Alpha MOS). The electronic tongue was equipped with a 16-position autosampler, an automatic stirrer, and an Ag/AgCl reference electrode. The assay was performed using amounts equivalent to 40 g pulverised meat dissolved into 160 mL distilled water, homogenised for 30 s, and then filtered using syringe filter (0.45 μm). Operating conditions were as follows: 25 mL sample volume, 200 s acquisition time, 10 s cleaning time, 3 min per analysis, and 5 replicates per sample. The data were expressed as means and standard errors of the means.
Meat samples were analysed for moisture, protein, lipid, and collagen contents using a Food ScanTM Lab 78810 (Foss, Hilleroed, Denmark) according to the methods of the Association of Official Analytical Chemists (AOAC, 2000; Seo et al., 2015). The moisture content was measured from 5 g of meat, and samples were then dried in a conventional oven at 105°C and 100 mm Hg for 16 h. Crude FCs were determined by the Soxhlet method with petroleum ether, and protein contents were estimated using the Kjeldahl method. In addition, collagen content was assessed by calculating the hydroxyl proline content (Samuel, 2009). The samples and hydroxyl proline standards were evaluated by measuring the absorbance at 558 nm using a spectrophotometer.
The pH values were determined using a pH*K 21 (NWK-Technology, Lengenfeld, Germany) on the surface of the meat. Meat color also was measured using a Minolta Chroma Meter CR-400 (Minolta Camera, Tyoko, Japan). Color was recorded as lightness (L*), redness (a*), and yellowness (b*).
WHC was determined as previously described. First, a 2-mL filter was weighed and then weighed again after placing 500 mg ground sample in the upper filter of the centrifuge tube. The surface area of the meat and the total area were measured using a planimeter (Type KP-21, Koizumi, Tokyo, Japan).
The peak areas of metabolites were subjected partial least squares-discriminant analysis (PLS-DA; SIMCA version 14; Umetrics, Umea, Sweden) to visualize cluster separation between high- and low-quality groups. The statistical significance (p<0.05) of metabolite concentrations, sensory and taste evaluation, and chemical compositions was evaluated by unpaired t-tests for meat quality. To obtain meat metabolic profiles, NMR spectra were binned with a 0.001-ppm binning size. The binned spectra were normalised to the total area and aligned using the icoshift algorithm of MATLAB R2013b (Mathworks, Natick, MA, USA). The binning results were imported into SIMCA 14.1 (Umetrics). PLS-DA was performed on Pareto-scaled data to visualise general clustering of all samples on the scores plot, which was defined with 95% confidence intervals. Variable importance in projection (VIP) plots were also utilised as potential indicators (Table 1). VIP values greater than 1.0 were considered important in discriminating between groups. Data analysis of the metabolites and physicochemical characterization were performed using Excel 2016 (Microsoft) and GraphPad Prism ver. 5.03 (GraphPad Software).
Results
Meat metabolome profiling using NMR was performed between high- and low-marbled meats, as shown with regard to differences in intramuscular fat accumulation (Fig. 1A). PLS-DA score plots revealed distinct clustering of the meat quality based on qualitative and quantitative data (Fig. 1B). Several metabolomics data were quantified, with missing data of 4%–12%. The characteristics of the PLS model were sufficient, as follows: NMR (R2X=0.435, R2Y=0.726, and Q2=0.646). PLS-DA loading plots showed differences in metabolite concentrations between beef quality attributes (data not shown). A total of 28 metabolic compounds from the beef samples were identified by using NMR. Data sets were validated by cross-validated analysis of variance and the permutation test (n=200, PLS-DA validation plot; Fig. 1C). N,N-Dimethylglycine, creatine, lactate, carnosine, carnitine, sn-glycero-3-phosphocholine, betaine, glycine, glucose, alanine, tryptophan, methionine, taurine, and tyrosine were representative metabolites in between high- and low quality meat. Thus, these metabolites could be representative of different marbled groups in this study. The selected metabolites, including N,N-dimethylglycine, creatine, glucose, and lactate, etc., show increased levels in the high-quality group (p<0.05; Fig. 2).
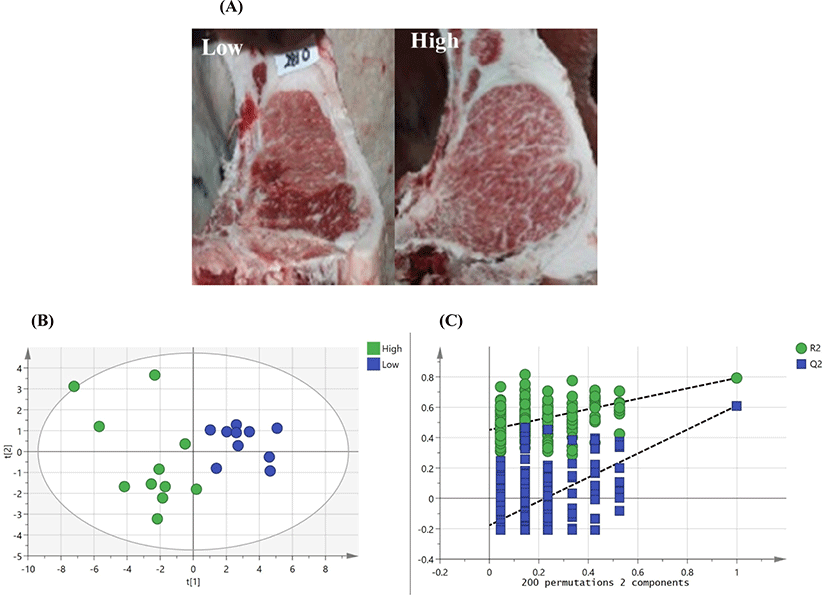
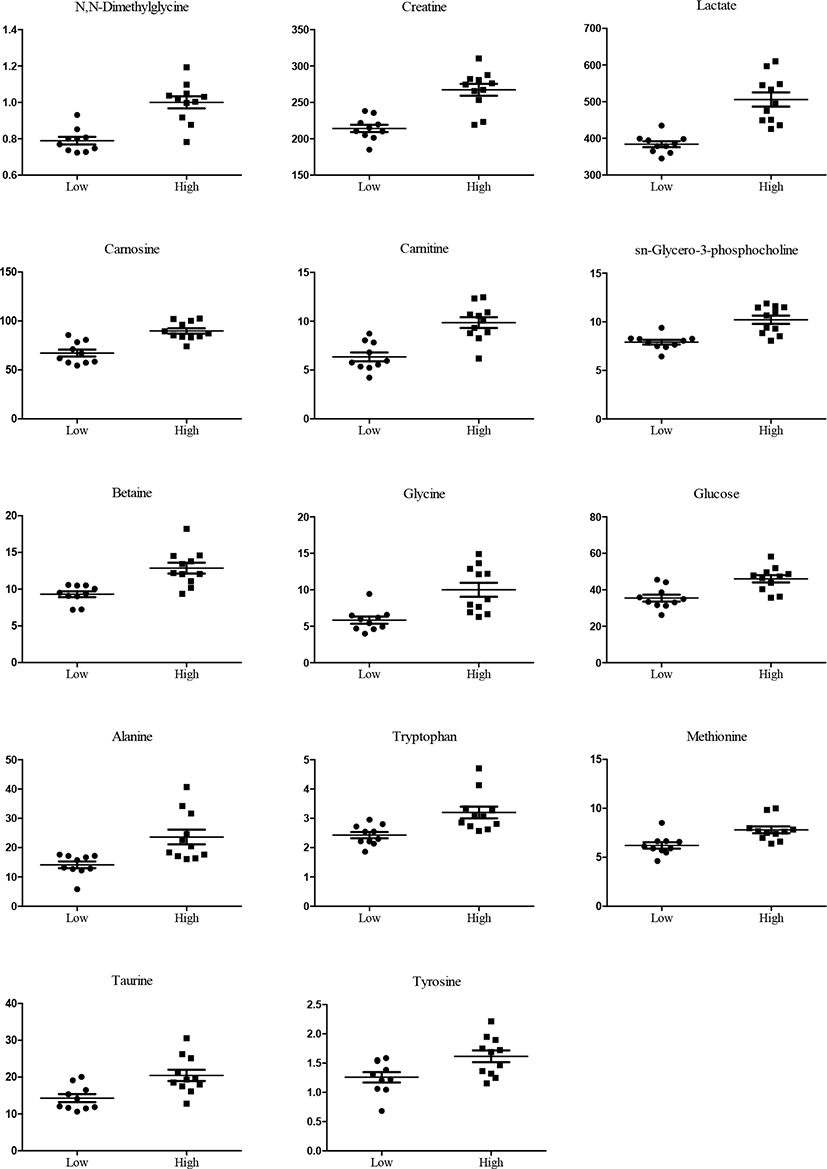
PCA of high- and low-marbled meats and metabolite set enrichment analysis (MSEA) were performed to assess patterns of changes in various metabolic pathways for predicting important metabolic pathways. To predict meaningful metabolic pathways, enrichment analyses for 28 selected metabolites were performed based on VIP scores by NMR (Fig. 3). Protein biosynthesis and glycine, serine, and threonine metabolism were contributed in 28 selected metabolites using MSEA analysis [p<0.01, false discovery rate (FDR)<0.05]. Based on these findings, metabolic pathways affecting meat quality were ranked as follows based on enrichment in the high-versus low-marbled groups: protein biosynthesis>betaine metabolism> methionine metabolism>glycine, serine, threonine metabolism>urea cycle>glucose-alanine cycle>alanine metabolism (p<0.001, FDR<0.001). Thus, MSEA was used for searching the potential biomarkers more than general statistics.
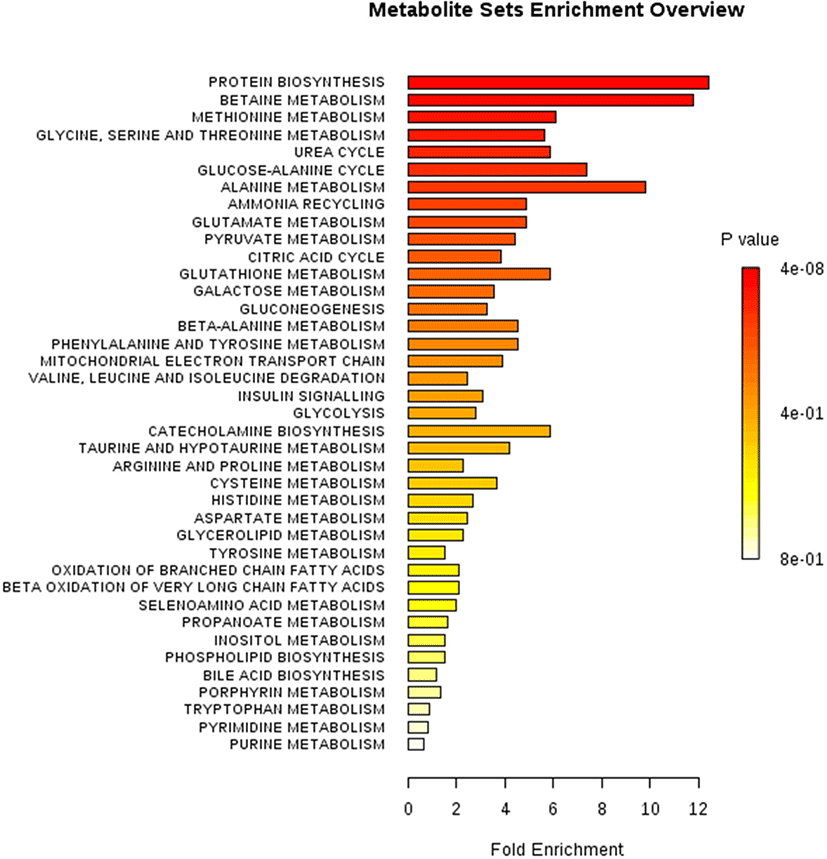
Physicochemical and taste scores were determined between two marbled groups (Table 2). Improved color (p<0.05), flavour (p<0.05), juiciness (p<0.001), and tenderness (p<0.001) were determined in the high-marbled group. Sensory results showed higher scores in the high-marbled groups than these of low-marbled groups. Taste score (e.g., saltiness, umami, and sweetness scores) were higher in the high-marbled meat using an electronic tongue (p<0.05). In contrast, there were no significant differences in sourness and bitterness scores between two marble groups. Moisture and FCs were increased in high-marbled meats (p<0.001). No significant differences were observed in protein and collagen contents Lightness (p<0.001) and yellowness (p<0.01) values were significantly increased in high-marbled groups. The high-marbled groups showed lower shear force values compared with the low-marbled groups (p<0.05). The range of meat shear force obtained in this study was 33.73–49.02 N. The WHC did not differ significantly between the high- and low-marbled groups.
Discussion
Analysis of metabolomics data has improved our understanding of metabolic networks and biological systems. In this study, beef quality attributes and taste compounds are contributed to metabolomics profiles. We estimate metabolomics analysis whether metabolites in beef quality attributes were affected levels of taste compounds, which is consumers require more information.
Our results showed that 28 metabolites were identified from 21 meat samples using NMR. The metabolites based on beef quality attributes were integrated with sensory, genomics, proteomics, and metabolomics by various methods (Carrillo et al., 2016; Jiang and Bratcher, 2016; Kodani et al., 2017; Picard et al., 2015). Thus, multivariate analysis used to elucidate a more detailed evaluation of two marbled groups. The levels of taste-related compounds, color, and sensory characteristics were also partly increased in high-marbled meat compared with that in low-marbled meat. These findings suggested that some metabolites such as sour-salty (e.g., lactic acid), sweet (e.g., alanine and glycine), bitter (e.g., creatine), and miscellaneous substances (e.g., methionine, carnosine, taurine) were related to taste differences between in high- and low-marbled groups.
The moisture content and FC of meat is affected by animal type, age, sex, feed, and muscle location and function (Nian et al., 2018; Seong et al., 2016). Young cattle have higher water levels because collagen, protein, and fat in the meat have not fully developed. Protein content is influenced by dietary factors before and during slaughtering. The high protein content of meat causes increasing WHC and decreased free water contents (den Hertog-Meischke et al., 1997; Qiaofeny et al., 2008). The average moisture content and FC of meat in this work ranged from 58.47% to 63.65% and 11.60% to 16.85%, respectively. Young animals have a higher moisture content than older animals due to increased intramuscular fat deposition in meat, accompanied by decreased water content (Ueda et al., 2007). In this study, moisture content and FC are negatively measured between two marbled groups in accordance with the accumulation of fat in muscle.
Generally, NMR analysis provides comprehensive information on glucose, amino acids, pyruvate, lactate, and other small molecules involved in numerous metabolism pathways. Here, glycine and serine metabolism, glutamate metabolism, and betaine metabolism, including betaine, creatine, dimethylglycine, alanine, creatine, methionine, glutamate were observed in high-and low-marbled meat. Therefore, the biosynthesis and degradation of proteins may differ according to beef meat attributes.
The main components affecting meat taste are chemical compounds (Bu et al., 2013). However, because many metabolites contribute to palatability, accurate prediction of taste-associated metabolites is not easy. Additionally, the metabolite composition of meat can differ owing to the quality of meat, causing changes in flavour. Meat taste is commonly a combination of five taste traditional sensations, and palatability plays a major role among them. Especially, umami tastes come primarily from free amino acids, glutamic acid, and aspartic acid, and from certain 5-ribonucleotides such as IMP, guanosine-5-monophosphate (GMP), and adenosine-5-monophosphate (AMP) (Cambero et al., 1992; Kurihara, 2015; Pal Choudhuri et al., 2015; Rotola-Pukkila et al., 2015). Palatability also arises from the synergistic effects of glutamate and free nucleotides (Yamaguchi and Ninomiya, 2000). Binding of glutamate to taste receptors in the tongue results in umami sensation, and its intensity is significantly enhanced by the presence of free nucleotides, such as IMP, AMP, and GMP (Mouritsen and Khandelia, 2012). The taste intensity of nucleotides alone is weak (Kurihara, 2015; Yamaguchi and Ninomiya, 2000). However, the major nucleotide in meat is IMP, which further degrades to inosine, ribose, and hypoxanthine (Tikk et al., 2006). In this study, umami related metabolites were not significant differences as described above. However, it was higher in high-marbled groups using electronic tongue (p<0.05).
Notably, we found that glycine associated with sweetness was significantly increased in high-marbled groups (p<0.01). Glycine stimulates the release of dopamine and acetylcholine from tissue (Hernandes et al., 2007), and increased levels of glycine were also observed in plasma (Schmidt et al., 2016), consistent with changes in glycine levels of beef observed in this study.
Creatine is a key compound that plays important roles in muscle energy metabolism (Wyss and Kaddurah-Daouk, 2000). Increased creatine content in muscles may delay postmortem lactate formation and decrease in pH, potentially improving the WHC (Nissen and Young, 2006). However, lactate formation and pH levels were not significantly different between high- and low-marbled meat, except for the WHC.
According to Susumu et al. (2020), described metabolomes with meat quality traits, metabolomics is used for the exploration to searching key compounds contributing to the physicochemical properties and sensory evaluation scores, and thereby it contributes to accounting for meat palatability and quality traits. In this study, taste by electronic tongue were higher saltiness, umami, and sweetness in the high-marbled groups than these of low-marbled groups. The relevance of the electronic tongue for more rapid and sensitive screening of meat taste has become important. Additionally, the palatability in beef also is generally attributed to tenderness, flavour, and/or juiciness. Therefore, in future studies, quantification of these potential biomarkers may have applications in the prediction of beef quality attributes and taste compounds during the growing and fattening stages. Metabolites may act as good biomarkers for these parameters. Nevertheless, we still have limited knowledge of the roles of metabolites and their regulation in beef quality and taste.
Conclusion
NMR analysis was performed to identify the metabolic biomarkers in high- and low-marbled meats. Among 28 estimated metabolites, fourteen metabolites showed significant changes in the beef quality attributes. In this study, key metabolites related to palatability (umami) taste score, including glutamate and aspartate, were not changed between in low-and high-marbled groups using NMR analysis. Sweetness, sourness, and bitterness in high-marbled meat were high levels compared with low marbled meat using electronic tongue and NMR analysis. The use of the electronic tongue for evaluating meat taste scores also improves our understanding of appropriate combinations of taste-related metabolites for developing high marbling. Our finding suggested that metabolites could serve as potential biomarkers of MS-related taste. However, further studies are needed to confirm these findings.