Introduction
Response surface methodology (RSM) is a set of statistical techniques for modeling and optimizing responses through the design and analysis of experiments (Myers et al., 2009), which has been widely used in engineering, agriculture, life science, microbiology and food sciences. A search by Google Scholar revealed that the number of scientific articles whose titles mentioned ‘response surface’ was 157 in 2000 but it became 1,860 in 2018, which is an 11.8-fold increase during recent 18 years. This indicates that RSM has been established as an important tool for modeling and optimization in experimental sciences including food sciences of animal resources.
In RSM, the central composite designs (CCD, Box and Wilson, 1951) have been most frequently used as experimental plans, and the second-order polynomial regression models have been usually employed for data analysis. And, the response surface model can be said to be well fitted and reliable when it satisfies the following criteria: (1) the model is significant (the model p-value≦0.05), (2) the lack of fit is non-significant (the lack-of-fit p-value>0.05), (3) the r2≧0.9 (Giunta, 1997), and (4) the adjusted r2≧0.8 (Myers et al., 2009).
However, in reality, it is observed that, for some data, the analysis models, which are the second-order models in most cases, do not satisfy the above criteria. A remedy in this case is to use a third-order model that consists of linear, quadratic, cubic, and relevant interaction terms (Rheem and Rheem, 2012). For example, when there are two factors, letting X1 and X2 denote coded factors, the third-order model has the following terms: linear terms X1 and X2, quadratic terms X12 and X22, cubic terms X13 and X23, and the two-factor interaction term X1X2. There exist the cases where this method improves the model. But, this method is applicable to a CCD that uses five values, which are denoted by −α, −1, 0, 1, and α, as the levels of coded factors.
When the experimental design is a CCD in which −1, 0, and 1 are the levels of coded factors, as in Table 1, cubic terms cannot be added to the model, since (−1)3=−1, (0)3=0, and (1)3=1, which makes the cubic terms equal to the linear terms. For example, in Table 1B, we can see that X1=X13 and X2=X23, and thus the X13 and X23 terms cannot be chosen from among the candidates of additional model terms for augmenting the second-order model.
This problem can be solved by adding the terms of the interaction between the linear term of one factor and the quadratic term of another factor. For example, in Table 1B, X12X2 and X1X22 are such interaction terms. The model that contains such interaction terms can be named a heterogeneous third-order model, since the sum of the exponents in each of such interaction terms is three. Thus, a remedy in this case is to augment the second-order model to the heterogeneous third-order model by adding the X12X2 and X1X22 terms, which are chosen from among the candidates of additional model terms in Table 1B, to the second-order model.
A dataset, which is obtained through the screening of the data in Ahn et al. (2017), will be re-analyzed for the illustration of the remedy suggested in this research note. Since Ahn et al. (2017) has two responses and a purpose of it is the multi-response optimization of them, this research note, which is a continuation of Rheem and Oh (2019), will model both responses by using heterogeneous third-order models, and optimize them simultaneously by employing the desirability function technique.
Materials and Methods
Data analysis should include data screening, which is necessary for accurate modeling. The original data to be used for re-analysis is the data described in Ahn et al. (2017), in which they tried to optimize manufacturing conditions for improving storage stability of coffee-supplemented milk beverage by using RSM. Through data screening, one outlier was deleted from their data (Rheem and Oh, 2019). The response variables, Y1 and Y2, and the factors in this experiment are described in Table 1A. The dataset from which an outlier is eliminated is given in Table 1B. Here, the experimental design is a CCD for two factors with the coded levels of −1, 0, and 1. Using this data, we will fit to the data second-order models and heterogeneous third-order models.
Results and Discussion
First, for each of Y1 and Y2, the second-order polynomial regression model containing 2 linear, 2 quadratic, and 1 interaction terms was fitted to the data by using RSREG procedure of SAS/STAT.
For both Y1 and Y2, the second-order models are unsatisfactory. For Y1, the model is non-significant (p=0.5962), the lack of fit is significant (p=0.0131), the r2=0.40<0.9, and the adjusted r2=−0.11<0.8. Also, for Y2, the model is non-significant (p=0.2924), the lack of fit is significant (p=0.0203), and the r2=0.57<0.9, and the adjusted r2=0.21<0.8. None of the four criteria are met for both Y1 and Y2. Thus, we will augment the analysis models for their improvement.
For each of Y1 and Y2, since the second-order model has a poor fit for the data, next we will fit to the data a heterogeneous third-order model that consists of the X1, X2, X12, X22, X1X2, X12X2, and X1X22 terms, by adding the X12X2 and X1X22 terms to the second-order model, in the anticipation of a possible improvement in modeling.
For both Y1 and Y2, the heterogeneous third-order models are satisfactory. For Y1, the model is significant (p=0.0243), the lack of fit is non-significant (p=0.1276), the r2=0.94>0.9, and the adjusted r2=0.84≧0.8. Also, for Y2, the model is significant (p=0.0371), the lack of fit is non-significant (p=0.0820), and the r2=0.93>0.9, and the adjusted r2=0.80≧0.8. All of the four criteria are satisfied for both Y1 and Y2.
Thus, we accept these models as our final models. Letting and denote the predicted values of Y1, and Y2, we specify our heterogeneous third-order models as
and
where the coefficients b1, b2, …, b122 and c1, c2, …, c122 are given in Table 2A and Table 2B.
Each of the three-dimensional (3D) response surface plots was drawn with the vertical axis representing the predicted response and two horizontal axes indicating the two explanatory factors. Fig. 1A and 1B are the 3D response surface plots for the effects of the two actual factors on the two predicted responses.
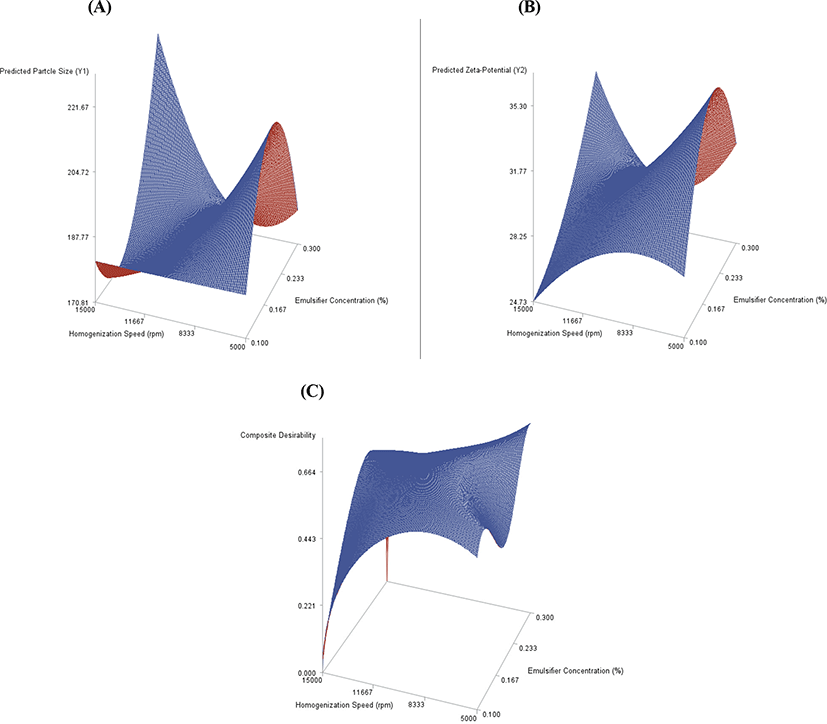
In Ahn et al. (2017), the optimization objective was to minimize Y1 (particle size) and maximize Y2 (zeta-potential) simultaneously. For this multi-response optimization, we modified the desirability function technique of Derringer and Suich (1980). In this modified technique, first, we define the desirability function for the minimization of Y1 as
and define the desirability function for the maximization of Y1 as
Here, for , when is minimized, D1 becomes 1; otherwise 0≦D1<1, and for , when is maximized, D2 becomes 1; otherwise 0≦D2<1. Now, we define CD, which means the composite desirability, as
which is the geometric mean of D1 and D2. Then, we find the combination of the values of X1 and X2 that maximizes CD. This combination is the optimum point of (X1, X2). Now, by converting this optimum point to the combination of the levels of the actual factors, we achieve the multi-response optimization of minimizing Y1 and maximizing Y2 simultaneously.
For the minimization and maximization of Y1 and Y2 and the maximization of CD, we performed the searches on a grid (Oh et al., 1995). First, we obtained Minimum ( )=170.8131135, Maximum ()=221.6698750, Minimum ( )=24.7334750, and Maximum ( )=35.2957228, and using these values, we implemented our modified desirability function technique that maximizes the composite desirability defined above. Fig. 1C shows the 3D surface plot of the composite desirability function for our multi-response optimization. Table 2C presents the results of our multi-response optimization.
In Ahn et al. (2017), at their optimum point, their Y1 value was 190.1 and their Y2 value was −25.94±0.06, whereas, at our predicted optimum point, the predicted Y1 was 183.4 and the predicted Y2 was 30.93. We can see that our predicted minimum of Y1 is smaller than their observed Y1, and our predicted maximum of Y2 is greater than their observed Y2. Their optimum conditions for their multi-response optimization were F1=speed of primary homogenization (rpm)=5,000 and F2=concentration of emulsifier (%)=0.2071, whereas our optimum conditions are F1=5,000 and F2=0.295. We can see that our predicted combination of optimum factor levels is different from theirs. A validation experiment will be needed to verify the result of multi-response optimization obtained by the method proposed in this article.
Conclusion
This article suggests the use of the heterogeneous third-order model for better modeling and a modified desirability function technique for multi-response optimization. The heterogeneous third-order model can be used when (1) the experimental design is a two-factor central composite design having −1, 0, and 1 as the coded levels of factors, and (2) a second-order model fails to provide a good fit for the data. How to construct the heterogeneous third-order model is to use X1, X2, X12, X22, X1X2, X12X2, and X1X22 as model terms. A modified desirability function technique first defines a desirability function for each response according to each optimization objective, and then finds out the combination of factor levels that maximizes the geometric mean of the values from desirability functions for multiple responses. An illustrative new analysis of the data from a previous research has produced statistical models with better fits and optimization results with better predictions. This suggestion is expected to help enhance the quality of response surface analyses of the experiments in food science of animal resources.