Introduction
In several parts of the world, meat processing industries are facing cases of meat adulteration due to continuously mounting prices of meat, augmented demand and escalating trade at international level (Nunes et al., 2016; Spink et al., 2017). Previously one of the prominent case under discussion was the “horsemeat scandal”, in which burgers of minced beef were mixed with pork and horse meat. The case was firstly reported in the Ireland and the United Kingdom (UK), and then observed in 13 other countries of Europe (Premanandh, 2013). Chicken is the most common edible meat in the world because its cost is relatively low compared to other animals such as cattles (Vasconcelos et al., 2014). Therefore, the inclusion or substitution of low value items (such as chicken instead of high value beef) in meat products to increase financial returns is a potential adulteration problem. Economically motivated processed beef adulteration can be done by substituting low price meat element (pork, chicken) or adding extra amount of the plant based proteins. Processed and minced meat products are more likely to adulterate compared to fresh meat, because grinding, processing and using other ingredients can mask the effects of adulteration. The problem of finding the adulteration of processed beef usually becomes more difficult after heating process as heating results in denaturation of protein and disappearing of the meat chemical bonds (López and Alegre, 2009) which makes detection method more challenging. Thus, it becomes critical for many food buyers to find out the correct ingredient information. As such, the ability to recognize the fraudulent food products to guarantee the quality, safety and other characteristics of food is assertive to build and maintain customer confidence. This puts consumers at a great disadvantage as the intake of certain types of food is opposed by cultural and religious barriers e.g., Muslims and Jews do not eat pork because of their religious reasons (Abbas et al., 2018; Al-Kahtani et al., 2017; Boyacı et al., 2014; Zhao et al., 2014). Therefore, prevention of meat adulteration, proper labeling on food and prevention of unfair competition in the meat sector have become an important issue for the competent authorities of the meat sector (Alamprese et al., 2016; Kamruzzaman et al., 2015).
Many classical investigations have documented the efficacy of different analytical methods for providing comprehensive results. Among such techniques, polymerase chain reaction (PCR) is a well-known molecular method which involves chemical amplification of specific DNA sequences (Ha et al., 2017). This technique is very useful for the identification of different species in a mixed meat sample or detection of meat origin based on specific DNA sequences that are indigenous to different meat species (Jira and Münch, 2019). Chromatographic, immunological and electrophoresis are the common means of detecting foreign proteins mainly soybean proteins (López and Alegre, 2009). Hoffmann et al. (2017) explored the ability of HPLC –MS/ MS screening method for simultaneous detection of plant protein like (soy, pea, and lutein) in meat products. Even though the above described methods offer high accuracy and show resilient robustness, they still have numerous shortcomings e.g., these are more expensive, laborious and time-consuming and there is need for expert to perform the experiment and fairly long time needed for preparation of sample and performing analysis. Hence, there is a need for developing rapid and accurate techniques appropriate for at-line authentication and easy to be applied by governmental agencies and (QA) quality assurance engineers for tracking adulteration in processed products of meat which has pushed efforts to introduce fast, innovative and reliable detection techniques to ensure authenticity, quality and safety of meat and meat products (Aït Kaddour et al., 2018; Alexandrakis et al., 2008; Cawthorn et al., 2013).
Spectroscopic techniques are useful for the determination of meat quality and authenticity because these are more rapid and non-destructive techniques (Troy et al., 2016; Xiong et al., 2017). Relatedly, spectroscopic methods, in combination with chemometric tools, have demonstrated their potential to detect meat authenticity and determined quality in a consistent manner. For instance, several investigations have examined such as Fourier transform infrared (FTIR), near infrared spectroscopy (NIR) and mid infrared spectroscopy (MIR) as means of measuring meat quality (Rady and Adedeji, 2018; Rahman et al., 2017; Yang et al., 2018). Similarly, fluorescence spectroscopy has demonstrated its ability to detect various types of plants and animal-based adulterants in meat (Kartheek et al., 2011). Fluorescence spectroscopy is an inexpensive, simple, rapid, and relatively non-destructive analytical method. Recently, low cost, simple and accurate fluorimeter has been combined with advanced systematic software which gave rapid, consistent, simple, repeatable values and magnification of the spectra. Therefore, many fluorometric approaches have been developed to study the quality, and authenticity of food products (Danezis et al., 2016). Meat contains many fluorescent compounds known as intrinsic and extrensic. Some lipid oxidation products in meat are also fluorescent compounds mainly pyridinoline in collagen, protoporphyrin IX (Hassoun et al., 2019), tryptophan residues in protein and vitamin A in meat fat (Sahar et al., 2016). Combined effect of fluorescence spectroscopy and chemometrics has wide applications in authentication and classification of meat products (Sahar et al., 2016) storage, cooking and processing circumstances (Hassoun and Karoui, 2015), and in microbial spoilage (Aït-Kaddour et al., 2018). Quantitative assessments of some meat components (e.g., fat and fatty acids) were established by using multivariate regression methods (Aït-Kaddour et al., 2016). Similarly, fluorescence spectroscopy has also shown the capability to detect meat quality (Kartheek et al., 2011). Studies related to the use of fluorescence spectroscopy for detecting meat quality are primarily focused on measuring fluorescence of proteins (fluorophores; amino acids), collagen, vitamins, adipose tissues and nucleic acids. There are a lot of studies on meat adulteration that carried out through FTIR, NIR, MIR and Raman spectroscopy but no study is in our knowledge that has conducted on detecting meat adulteration through fluorescence spectroscopy. Accordingly, the present work aimed to explore the potential of fluorescence spectroscopy to check its sensitivity in determining meat adulteration in a rapid in raw minced meat samples. Graphical abstract has been presented in Fig. 1.
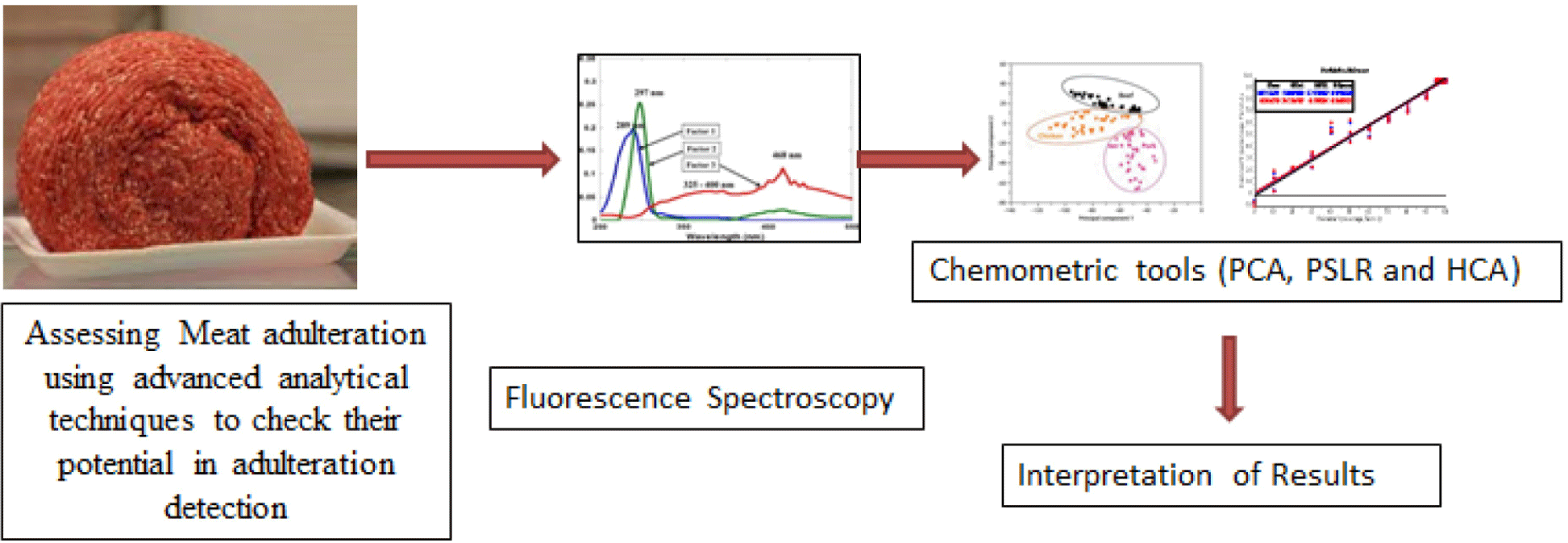
Materials and Methods
Three different batches of each fresh beef (chuck roast) and chicken thigh samples were randomly procured from local market then stored at 4°C before mincing, substitution, and performing analysis. Different levels of chicken meat were added to minced beef samples to make adulterated samples with three different batches of meat. Levels of adulteration ranged from (1%, 2%, 3%, 4%, 5%, 10%, 20%, 30%, 40%, 50%, 60%, 70%, 80%, 90%, and 100%) for beef adulteration with chicken to obtain 144 (3×3×16) blended samples included pure beef and chicken meat samples in total. These prepared binary meat samples were than homogenized by using laboratory homogeniser (laboratory T 25 digital ULTRA-TURRAX®, IKA, Königswinter, Germany) and stored at 4°C for performing analysis. Samples were analyzed for fluorescence spectroscopic analysis coupled with chemometric analysis to explore the sensitivity of technique in finding presence of adulterant level in minced beef meat. Three samples per level of adulteration were taken and all (pure and adulterated samples) were tested in triplicate. Flow chart of study plan has been presented in Fig. 2.
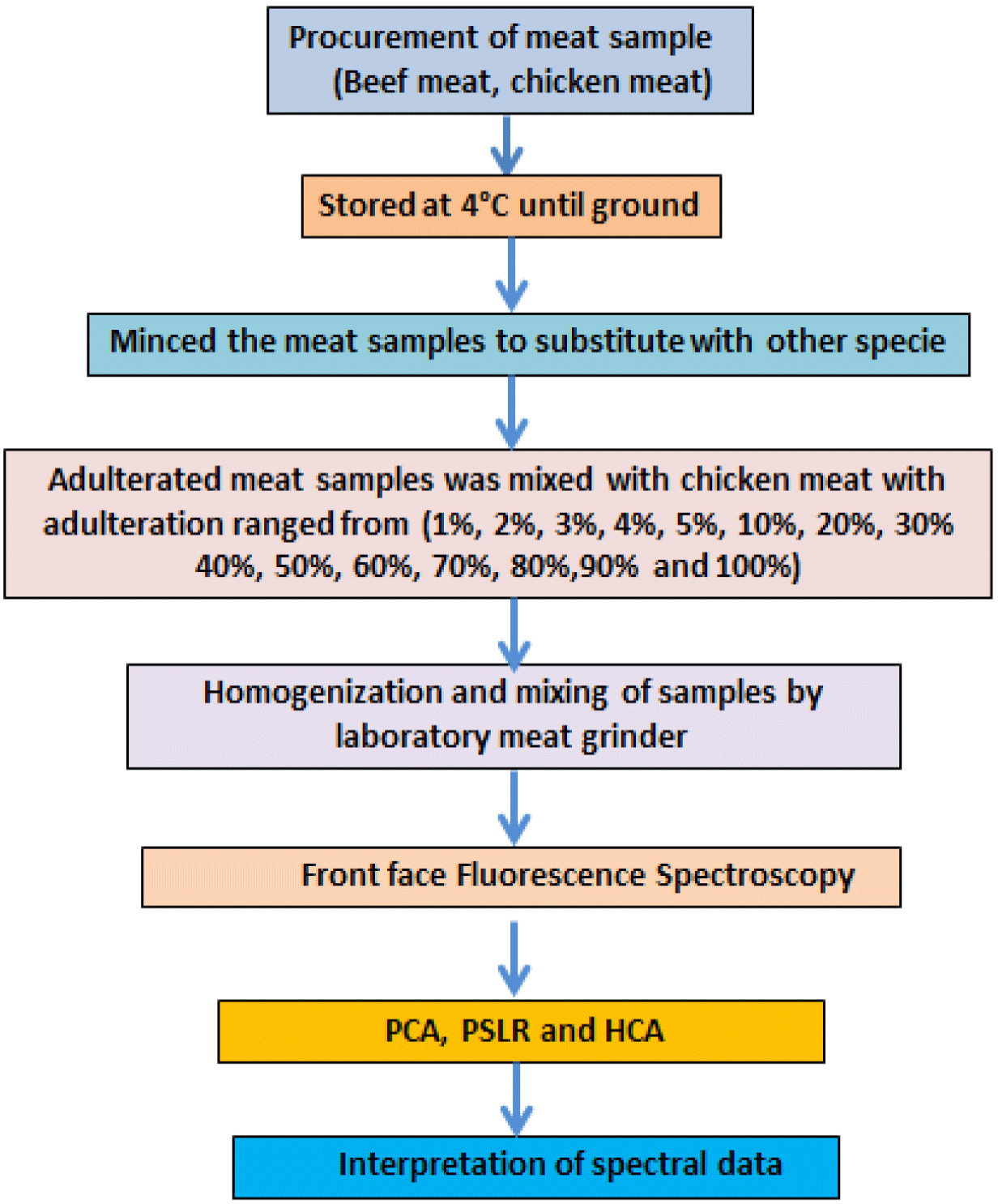
Fluorescence spectra were taken from all the meat samples and results were interpreted by applying chemometric tools to evaluate the potential of this analytical technique. For each spectrum, total 3 scans were taken and averaged so totally, 144 spectra were collected i.e., 3 (No. of spectra on individual sample) × 16 (Total number of independent samples for each treatment) × 3 (No. of replicates for each treatment) = 144.
Fluorescence emission spectra of meat samples were collected by fixing different excitation wavelengths (290 nm, 322 nm, 340 nm) using various emissions ranges (300–600 nm) while excitation spectra with fixed emission wavelength 410 and excitation range 252–370 nm. Spectra were taken on fluorescence spectrophotometer (Fluoro-Max 4, HORIBA, Piscataway, NJ, USA) mounted with a sample holder (Front-face). The angle of incidence for radiation was set at 56° and slit opening was adjusted in the range of 0.25–5.00 nm to minimize the phenomena of depolarization, light scattering and light reflection. All the spectra were recorded at room temperature and the experiment was conducted in three replicates for each treatment. The emission spectra were recorded with the excitation wavelengths set at 290, 322, and 340 nm, in the emission range of 305–450 nm, 340–550 nm, and 360–580 nm respectively, and the excitation spectra were acquired with the emission wavelength at 410 nm and excitation range 252–370 nm. These spectral ranges mainly related to the fluorescence of tryptophan, Vitamin A, nicotinamide adenine dinucleotide NADH, respectively, which previously used by (Aït-Kaddour et al., 2018; Sahar et al., 2009; Skjervold et al., 2003) and many others. Three (3) spectra were taken on each sample.
The Unscrambler X 10.5 software was used for chemometric analysis, to preprocess the fluorescence spectra for correction of the baseline. Prior to chemometric analysis the unwanted systematic variations (e.g., scattering effects, baseline shifts, and external factors) were removed. Spectra were pre-treated by applying Savitzky-Golay smoothing and normalized through Standard Normal Variate (SNV) correction for performing cluster analysis (CA) (Meza-Márquez et al., 2010).
PCA is a statistical procedure which is applied for converting the large number of potentially correlated factors into small number of uncorrelated factors known as principal components (Jolliffe and Cadima, 2016). In the current investigation, PCA applied to each offset of spectral data to draw similarity maps for noting the resemblances or variations among spectra taken from front face fluorescence spectroscopy (FFFS).
PSLR method is a type of predictive analysis and has been known as the most extensively employed statistical tool in many scientific studies (Vigneau et al., 2006). In this exploration PSLR analysis were applied on random mode on spectral data for cross validation of data to check relationship between adulteration level and spectral data. PSLR predictive analysis was performed in this study to predict level of adulteration in beef samples from fluorescence spectra because the predicted variables were characterized by a matrix (Bro, 1996). This analysis was applied to develop multivariate analysis model for adulterants detection i.e., chicken meat in ground beef meat.
The effectiveness of PSLR regression model is determined on the basis of its potential to correlate the spectral information with a matrix of the property of interest (level of adulteration), and to account for the factors which perturb the spectrum. PSLR analysis was applied to develop calibrated and predictive models and whole data were divided into calibration and prediction sets and cross validation was applied on the calibration set. In the current project, meat samples with different level of adulteration were used as calibration sets to build calibration models. The estimation of calibration performance of developed models was performed by calculating coefficient of determination for calibration (r2C) and root mean square error of calibration (RMSEC), whereas prediction performance of constructed models was evaluated by computing coefficient of determination for cross-validation (r2CV) and root mean square of error of cross-validation (RMSECV) (Vigneau et al., 2006).
CA was applied to find out the similarity among pure minced and adulterated meat samples. The results of CA are reported in the form of dendrograms that determine how the clusters merged hierarchically for all the spectrums of the samples obtained from the FFFS. Generation of dendrograms was performed by using Euclidean distance to calculate the sample similarities and to indicate the complete linkage clustering by Ward’s algorithm via unscramble software. Before Ward’s algorithm application the data were subjected to K-means and K-medians clustering which iteratively add or remove members from a set of clusters so as to minimize the sum of distances of cluster members to their cluster centers. These methods use less memory than hierarchical clustering methods and are therefore suitable for large data sets (Deniz et al., 2018).
Results and Discussion
Fluorescence Spectra were collected at fixed excitation wavelengths of 290 nm in the emission range of 305–450 nm, 322 nm with emission range of 340–550 nm, 340 nm having excitation wavelength range of 360–600 nm, and 410 nm with excitation spectral range of 252–370 nm.
Two-dimensional fluorescence emission spectra of beef samples adulterated with different percentages of chicken meat were collected at fixed excitation wavelength of 290 nm in the emission wavelength range of 305–450 nm (Fig. 3A), which were assigned for tryptophan content present in meat myofibril protein (Skjervold et al., 2003) and free amino acids in meat (Lawrie and Ledward, 2006). This wavelength served as fingerprint to evaluate meat tenderness and determination of microbial spoilage of beef (Aït-Kaddour et al., 2011; Aït-Kaddour et al., 2018), to classify different beef muscles (Dufour and Frencia, 2001; Gatellier et al., 2009; Sahar and Dufour, 2015; Sahar et al., 2009; Sahar et al., 2016) and for chicken breast filet characterization (Sahar et al., 2011). The result of this study showed fluorescent bands in the emission range of 340–400 nm. Changes in intensities of the peaks varied as the level of chicken meat incorporation in minced beef were observed. Pure chicken has highest peak at 375 nm and pure beef has lowest peak because of lesser intensity of absorption than chicken and adulterated samples. The adulteration level from 1%–5% chicken in beef showed overlapping on pure beef spectra while samples from 10% onward to 90% showed spectra with different intensities according to the presence of different levels of adulteration in beef meat. These changes in the intensities could be explained by changes in the protein-lipid, protein-protein or protein-water contacts, according to the findings of Karoui et al. (2006a) and Karoui et al. (2006b). Boughattas et al. (2019) used the same wavelength for adulteration detection in pure canned tuna fish and their adulterated mixtures with different percentages. These spectra of fish samples showed maximum wavelength located at ~373 nm which corresponding the maximum emission of tryptophan (Karoui et al., 2017).
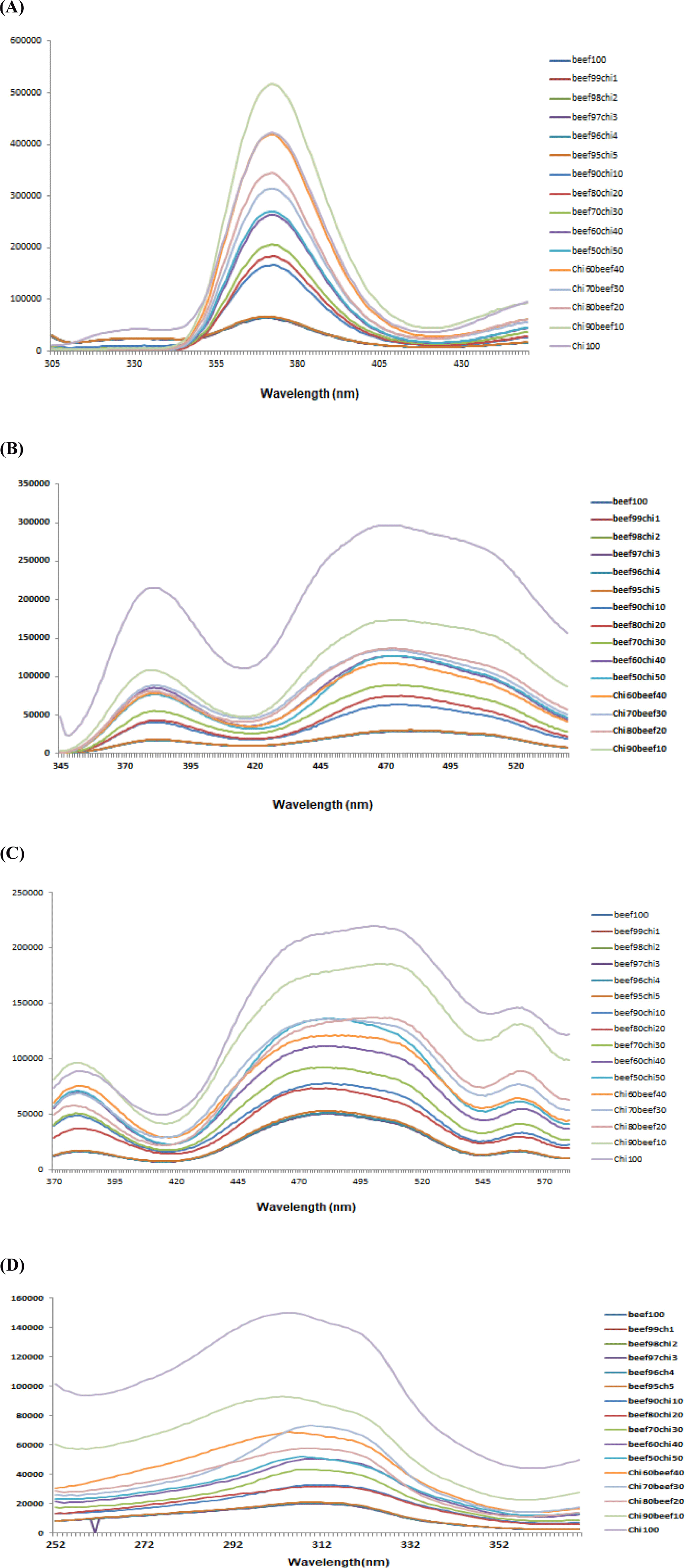
Fluorescence spectra at fixed excitation wavelength of (λex) 322 nm in the emission wavelength (λem) range of 340–550 nm also showed visual differences among spectra collected from pure minced beef, pure chicken and adulterated meat samples Fig. 3B. This excitation wavelength was assigned to vitamin A by Skjervold et al. (2003) and used by many researchers in their studies for meat muscles classification, fat determination in meat and for beef meat authentication (Aït-Kaddour et al., 2016; Aït-Kaddour et al., 2018; Sahar et al., 2016). Results of current study showed that maximum excitation of spectra were indicated as broaden spectra in wavelengths range from 430–530 nm and another excitation peak with lesser extent at 380 nm. Previously 380 nm has been shown as the best excitation wavelength for the quantification of connective tissue (Egelandsdal et al., 2002; Egelandsdal et al., 2005). Additionally, varied heights of peaks could be obtained due to the differences in the absorbance of fluorescent light. This difference is due to different concentrations of fluorophores based on changing percentages of beef in different adulterated samples which may be due to change in the fat percentage of beef and chicken meat.
Fig. 3C illustrates the fluorescence emission spectra of adulterated beef samples in the range of 360–580 nm at fixed excitation wavelength (λex) of 340 nm which are characteristic NADH present in beef and chicken. NAD(P)H is the coenzyme, which is universally present in living cells and considered a useful marker of the cell’s metabolic activity and carrier of electron and hydrogen. Visual observation of spectra collected from 100% minced beef, 100% chicken and adulterated beef samples with 10%, 20%, 30%, 40%, 50%, 60%, 70%, 80%, and 90% chicken showed clear differences in the heights and positions of peaks due to varied levels of adulteration while for 1%, 2%, 3%, 4%, and 5% no visible spectra were found. The prominent peaks were observed at 380 nm and maximum peak from “430–530 nm” with maximum at about 490 nm and one small peak at 560 nm. Pure chicken meat showed highest peak while pure beef showed lower peak. The peak height progressively increased as the addition of chicken in beef meat increase and trend of highness move in the sequence from low chicken percentage to high percentage and end with pure chicken peak. This wavelength was used by Boughattas et al. (2019) for determining adulteration in canned tuna fish and found maximum emission spectra at ~470 nm for canned tunas containing skipjack and 475 nm for canned tunas composed of bigeye (100%) and 478 nm for canned tunas for NADH. They demonstrated that the shape of NADH emission spectra depends on the composition of tuna cans.
Fig. 3D demonstrates the fluorescence spectra of beef samples incorporated with different levels of chicken meat at fixed emission wavelength of 410 nm in the range of 252–370 nm. Spectral differences among pure and adulterated beef samples can be seen with maximum absorbance of fluorescent light at emission wavelength of 310 nm with different heights. These variations in the heights of spectra reveal that fluorescence spectroscopy can be used to differentiate beef samples based on various percentages of chicken meat and useful information can be extracted from this spectral data by using multivariate analysis. Boughattas et al. (2019) assignd this wavelength for vitamin A for canned tuna fish to check the fish meat adulteration. Karoui et al. (2017) also assigned this emission wavelength to vitamin A in rapid differentiation of fresh and frozen-thawed sea fish fillets. Skjervold et al. (2003) identified vitamin A in 322 nm excitation and 440 nm emission bands in adipose tissues of meat as the fluorophore of a meat matrix. Meat fat contains vitamin A which due to its conjugated double bonds served as good fluorescent probe with fixed emission and excitation wavelengths at about 420 nm and 320 nm, respectively (Dufour and Riaublanc, 1997). In our study meat spectra at excitation wavelength (322 nm) showed maximum excitation at 380 and 480 nm while for fixed emission wavelength (410 nm) maximum peak observed at 320 nm. Sahar et al. (2009) said that due to the various fluoro-phores present in meat and difference in the composition of meat due to age, breed, envoirmental conditions and food, the collected spectra were most likely not form a unique pattern.
Thus, the spectral results of current research provide a baseline for using FFFS spectroscopy as a rapid, non-destructive and inclusive technique for identifying meat adulteration.
Graphical interpretation regarding the classification of minced beef samples adulterated with various percentages of chicken meat through PCA is illustrated in Fig. 4. For 290 nm spectral data 100% of the total variance was accounted for first two components of PCA in which PC-1 explained 99% of total variance while PC-2 described only 1%. Furthermore, PCA classified adulterated beef samples in to individual groups but with less clearance and dense overlapping was recorded for samples adulterated with chicken meat up to 95% and pure 100 % chicken samples showed scattering and the results weaken the model for classification of samples at 290 nm (Figure not given).
PCA similarity map for 322 showed that first two components are accounted for total 100% variance which showed the classification of beef meat adulteration with chicken meat with the adulteration level of chicken from (10% to 90%). The two-dimensional PCA for fixed excitation 322 elucidated that 100% of the variance was accounted for PC-1 while 0% was accounted for PC-2. Results proposed that PCA classified the meat samples into discrete groups based on substitution of beef with various levels of chicken meat and PCA for pure chicken meat was also showed in a separate group. The beef sample with 10%, 20%, and 30% chicken level are close to each other or overlapped and were near to 100% beef meat sample because of high level of beef meat 90%, 80%, and 70% in that adulterated samples. PCA plot didn’t make clear separation of samples containing 1%, 2%, 3%, 4%, and 5% chicken meat in beef meat (Fig. 4A). For 340 nm wavelength PCA results of FFFS spectral data regarding classification of beef samples based on different levels of adulteration are presented in Fig. 4B. Data reveled that PC-1 accounted for 98% of total variance. Additionally, PCA classified adulterated beef samples in to distinguished groups depending upon the percent incorporation of adulterants but scattering of samples weaken the classification results of PCA. While for (410 nm) PCA results showed satisfied classification in all the classes based on different levels of meat adulteration with chicken meat (Fig. 4C). PCA Data showed that 100% of the total variance was accounted for first two components of PCA. It was observed that PC-1 explained 99% of total variance while PC-2 described only 1%. Considering the classification, PCA did classify the data into all expected discriminant classes. Isolated groups were attained by samples adulterated with 10% to 90% chicken meat and the samples from 1%–5% chicken meat were overlapped with pure beef. 100% chicken meat samples showed scattered PCA score plots which described the poor potential of PCA to utilize spectral information for classifying pure chicken meat sample. However, generation of distinguished groups based on different levels of chicken meat in minced beef proposed that these spectra contain information that can be used for detection of beef adulteration with chicken meat which demonstrated the competence of fluorescence spectroscopy as a rapid and reliable screening method to detect minced beef adulteration by taking large data and large numbers of samples. Boughattas et al. (2019) and Karoui et al. (2017) applied PCA for all intrinsic fluorophores they used for fish meat adulteration detection and differentiation of fresh and frozen-thawed sea bass fillets. But for better classification they applied factorial discriminant analysis (FDA) to the first 5PCA of each intrinsic probe.
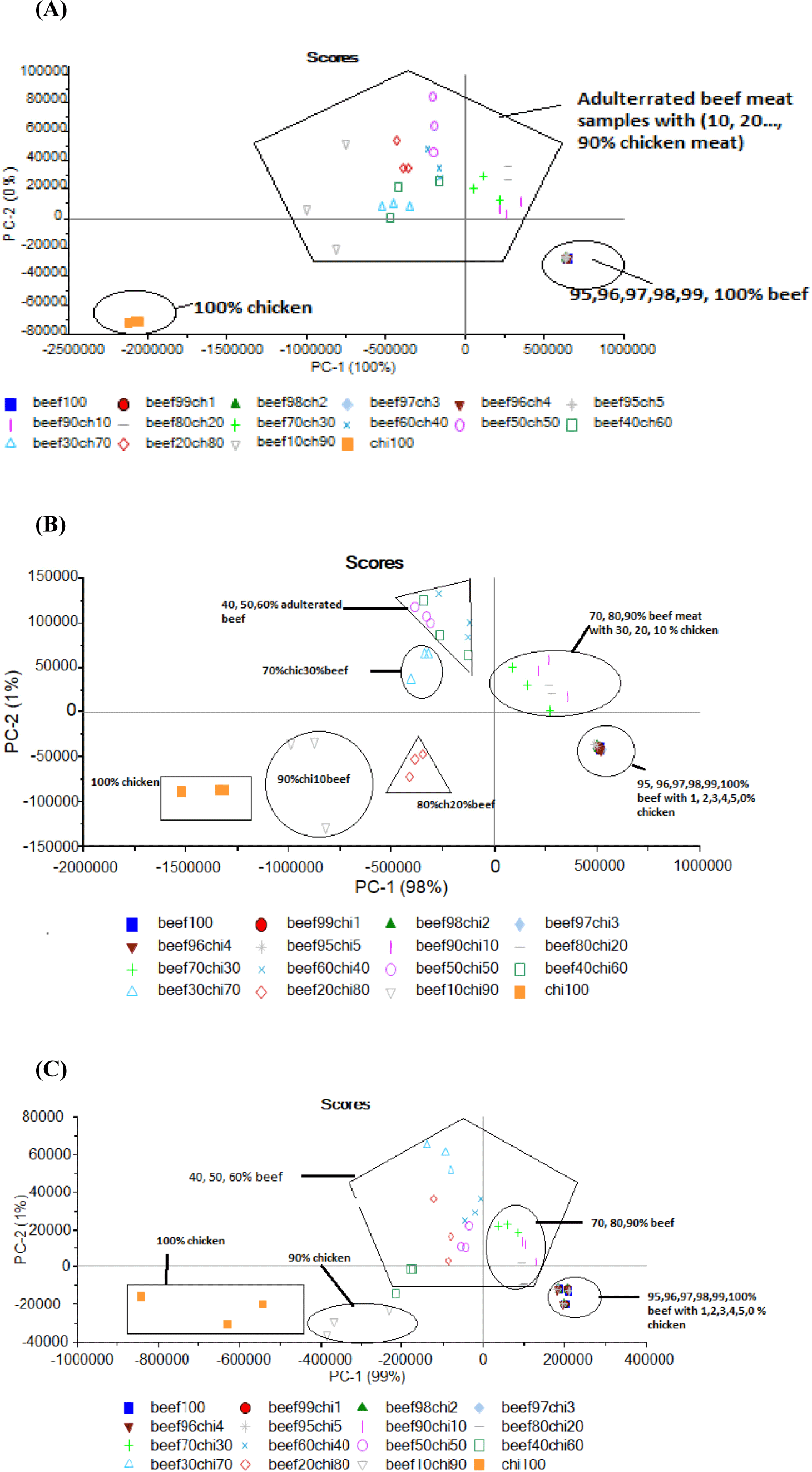
Findings of PLSR analysis based on adulteration level through fluorescent emission and excitation spectra have been presented in Table 1. Inferences proposed that moderate to good prediction models were developed describing effectiveness of fluorescent spectroscopy for predicting the adulteration. On the bases of RMSEC using cross validation the good calibration model was chosen. Best prediction model was selected by application of such model on the prediction data set based on (RMSECV) root mean square error (RMSE) of prediction and (r2) Regression coefficient of calibration and cross validation r2C and r2CV. Table 1 showed the PLSR results for predicting several adulterants’ level by selecting effective wavelengths.
Parameter | Fixed emission (nm) | Excitation wavelength range (nm) | r2C | RMSEC (%) | r2CV | RMSEP (%) |
---|---|---|---|---|---|---|
Level of adulteration (%) | 410 | 252–370 | 0.95 | 2.99 | 0.92 | 2.02 |
PLSR model were developed based on spectral data collected at fixed 290 nm for calibration and prediction measurements. The predictive models were obtained after preprocessing through normalization and mean centering of spectral data followed by development of calibration models based on r2 (regression coefficient) and RMSE values as by Egelandsdal et al. (2005). Regression results described that strong calibration model were developed for adulteration detection with high r2C values i.e., 0.89 for level of adulteration with poor RMSEC 3.49%. The results also showed that the good prediction model with r2CV value of 0.86 and poor root mean square error of calibration (RMSEP) value of 4.82% for level of adulteration. For 322 nm the developed calibration and prediction model for level of adulteration clearly indicated that predicted values were quite closer to the reference values. The coefficients of determination for calibration and prediction were 0.92 and 0.88 with corresponding moderate RMSEC and RMSEP values of 2.31%, and 1.99% respectively. PLSR prediction carried out at 290 and 322 nm revealed that strong prediction models with poor to moderate prediction errors were constructed explicating capability of fluorescent spectroscopy for predicting the adulteration in meat.
Validation of the developed calibration model for 340 nm exposed that coefficients of determination for prediction or validation (r2CV) were 0.95 for predicting level of beef adulteration and calibration (r2C) were 0.97 with less error values. While at fixed emission of 410 nm, validation of the developed calibration model showed that coefficients of determination for prediction or validation (r2CV) were 0.92 for predicting level of beef adulteration with chicken meat with moderate RMSEs for prediction i.e., 2.02 and calibration (r2C) were 0.95 with RMSEC 2.99%. These findings revealed that the model was good enough to differentiate minced beef samples based on different levels of adulterant incorporation. These inferences describe good efficiency of fluorescence spectroscopy coupled with chemometric analysis to predict the changes in the quantity attributes of beef under the influence of adulteration. Results for all probes presented in Table 1.
Egelandsdal et al. (2005) used front face fluorescence spectroscopy to detect the level and quality of connective tissues in beef and pork muscles and reported that fluorescent spectra along with PLSR modeling accurately identified various levels fluorescent molecules in both types of muscles and prepared sausage batters.
PLSR analysis confirmed that strongest model was developed at 340 nm wavelength for adulteration detection in the ground beef meat. Hence, this wavelength was further selected for validation of model by using a new external dataset with 10 adulterated samples. The obtained RMSEP value indicating the expected future prediction error value is quite less i.e., 0.09 which described good prediction efficiency. Additionally, highly strong correlation (0.95), r2-Pearson of 0.91, and standard prediction error of 0.06 was obtained which confirmed the prediction accuracy of constructed PLSR model based on used dataset (Fig. 5). Conclusively, this model can be effectively used for adulteration detection.
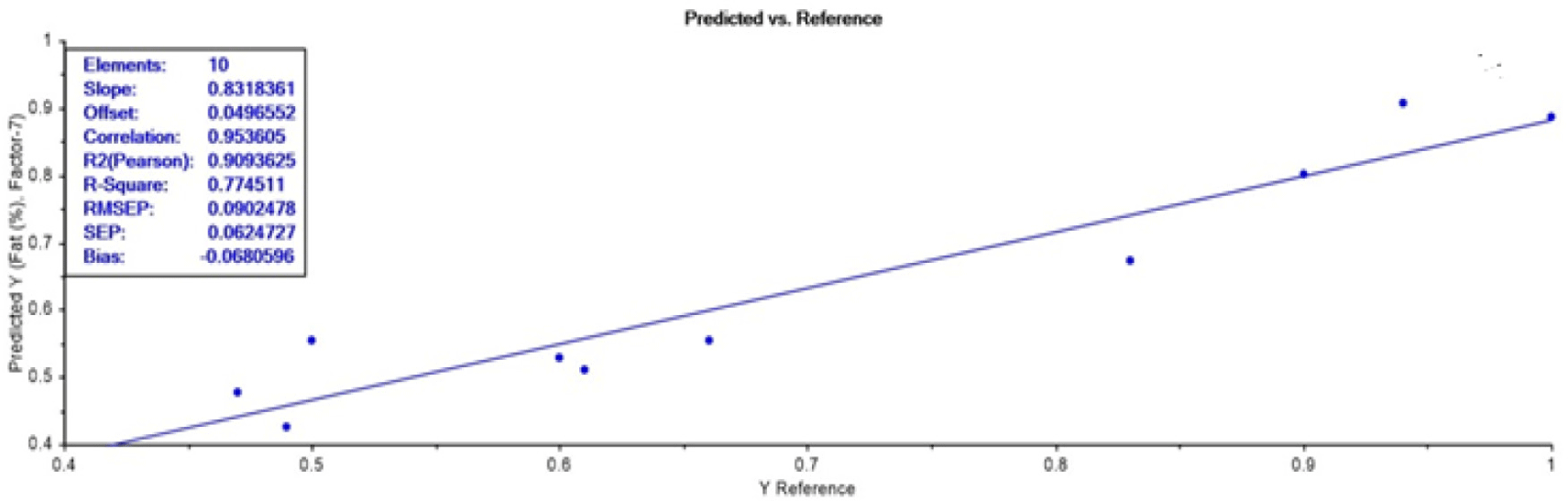
For CA, fluorescence spectra of pure and adulterated beef samples at fixed excitation wavelength of (λex) 290 nm in the emission wavelength (λem) range of 305–450 nm were taken. Binary meat mixture samples e.g., (99%, 97%, 95%, 90% beef adulterated with 1%, 3%, 5%, and 10% chicken meat and pure beef and chicken meat samples were taken for finding sensitivity and specificity of technique. The selected samples were preprocessed with SNV to minimize the noise and then subjected to K-means clustering to perform Hierarchical Cluster Analysis (HCA). K-means clustering divide data into grouping. For doing HCA, frequently used ward’s method was chosen by Deniz et al. (2018). The results showed that HCA classify the samples into ‘two clusters’ separately. In which 100% pure chicken meat was in separate cluster while adulterated sample in other cluster which further divided into different groups according to level of concentration. 90% beef 10% chicken in made separate group from other adulterated samples which having less adulteration level and comes in group with 100% beef e.g., 99% and 97% and 95% beef samples having 1%, 3%, and 5% chicken meat which indicates that HCA results detect the samples having 10% chicken which showed that fluorescence technique is sensitive for detecting upto 10% adulteration in beef meat at 290 nm wavelength.
At 322 nm results of fluorescence spectra of CA showed that samples of 100% chicken comes in separate cluster while adulterated samples in second cluster which further divided into groups containing 90% beef and 10% chicken, and 95% beef meat with 5% chicken meat were grouped into one group while 100% pure beef meat make group with 99% and 97% beef meat samples having 1% and 3% chicken meat as an adulterant in beef meat. CA of fluorescence spectra taken at 340 nm for detection of adulteration in beef meat indicates that two cluster were formed having one cluster with 100% chicken and second cluster having adulterated samples with three separate groups of one 90% beef 10% chicken and second group of sample with 95% beef and 5% chicken and third group contained 100% beef with 99%, and 97% beef. Thus the, results indicates that at 340 nm wavelength, fluorescence spectroscopy detect adulteration upto 5% concentration level. Result for CA of 340 nm showed in Fig. 6. CA of fluorescence spectra of adulterated beef samples at fixed emission wavelength of (λex) 410 nm in the excitation wavelength (λem) range of 252–370 nm were taken (99%, 97%, 95%, 90%, and 100% beef samples along with 100% chicken). The results showed that two clusters were formed containing one group with pure chicken meat samples and other group with beef 90% and chicken 10% while 100% beef formed group with 99%, 97%, and 95% beef samples. These findings indicates that HCA classified the meat samples having 10% chicken which showed that fluorescence technique is sensitive for detecting up to 10% adulteration in beef meat at 410 nm wavelength. Deniz et al. (2018) classified the adulterated meat samples by applying HCA to FTIR spectral data and found less good result with HCA for adulterated and pure sample classification in which they found 100% beef and 100% chicken spectra were well separated from each other by HCA, but a distinguished classification was not observed for the mixed beef-chicken meat samples. Figures for 290 nm, 322 nm, and 410 nm are not given.
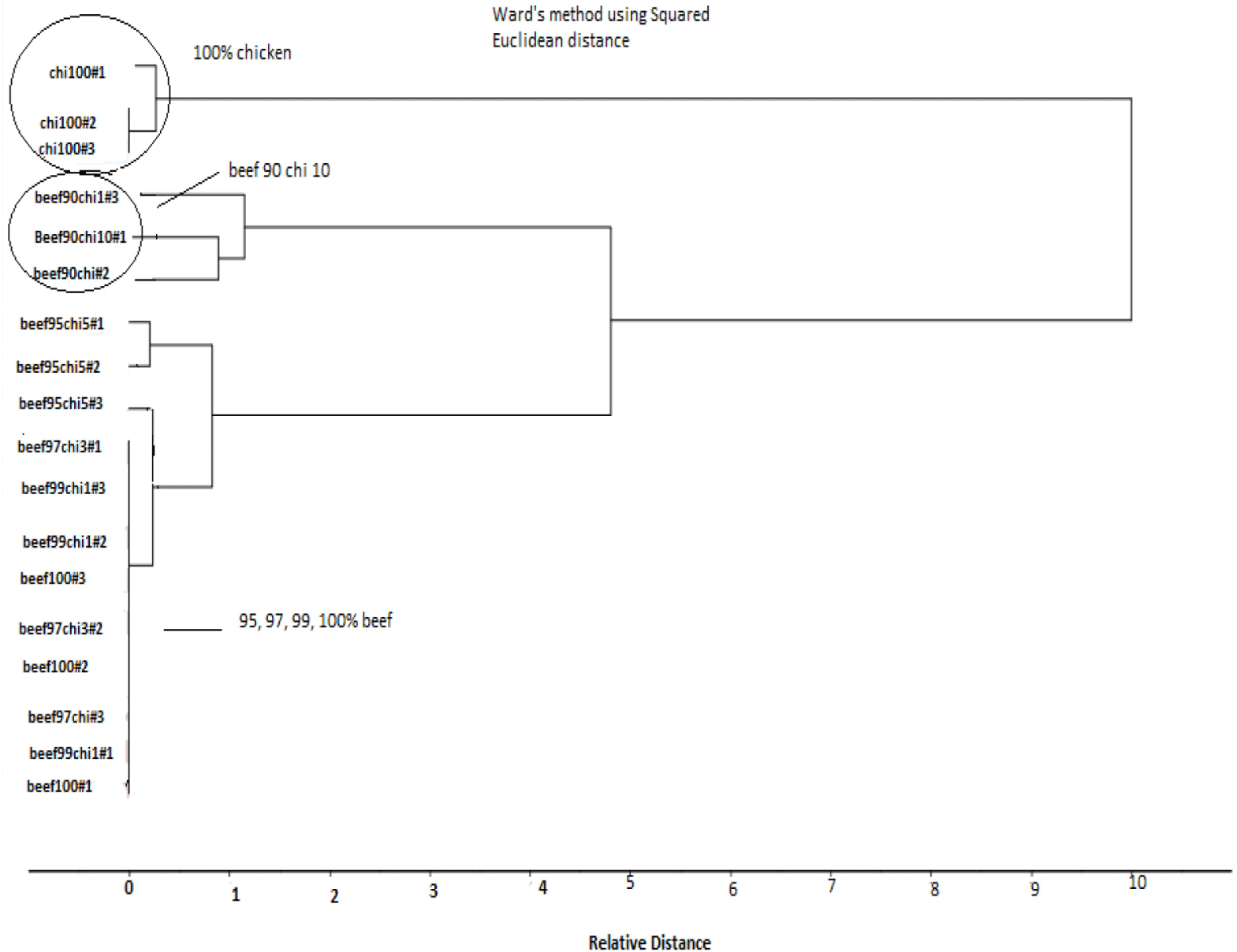
Conclusion
Meat quality and safety assurance is critical for both meat producers as well as meat consumers. This study has been conducted to explore the potential and sensitivity of front face florescence spectroscopy in tandem with mathematical modeling (PCA, PSLR, and HCA) for detecting adulteration in minced meat, as this technique is simple, fast and cost effective. The overall results showed the success rate of this method in determining adulteration level in meat up to 10%, but adulteration level from 1%–5% meat were indistinguishable. Results of PLSR models revealed that strong prediction of meat quality, authenticity and safety is possible even with a small number of PLSR factors. PCA and HCA results showed good classification of adulterated meat samples up to 10% which indicated that fluorescence spectroscopy could be developed into a rapid and reliable screening method to detect minced beef adulteration. Subsequently, more research work is needed with a large sample size and for intact muscles for establishing the utility of this spectroscopy for prediction minimum level of adulteration in meat. Comparing FFFS with other spectroscopic techniques (FTIR, MIR, and NIR) we can find technique that is more sensitive in detection of minimum level of adulteration.